Scaling smart energy solutions for complex environments with the C3 AI Platform
By Naoufal Layad, Senior Data Scientist, Mehdi Maasoumy, Vice President, Data Science, and Nevroz Sen, Manager, Data Science, C3 AI
Heating, ventilation, and air conditioning (HVAC) systems are an essential part of today’s modern building operations, ensuring optimal temperature, humidity, and air quality. While necessary for comfort, these systems also consume significant energy. Among their many components, air handling units (AHU) are crucial to regulating and circulating air, yet optimizing their performance is no small task — especially for complex environments such as hospitals, museums, and libraries.
With numerous variables at play — fan speeds, preheat levels, ambient conditions — achieving energy efficiency while maintaining comfort levels requires a sophisticated approach with a rigorous decision-making framework. The dynamic nature of these systems, coupled with changing conditions, makes data-driven solutions indispensable. Scaling such optimizations across large facilities introduces additional complexities, demanding robust computational and deployment capabilities — in particular, a unified AI platform.
In this blog, we showcase how C3 AI was able to quickly develop and deploy a data-driven optimization model for an operation-critical building, thanks to the platform services provided by the C3 AI Platform, including pipeline infrastructure and data, ML, and optimization tools. The solution elegantly combines advanced machine learning (ML) models with large-scale optimization, streamlining development, deployment, and monitoring across many buildings.
Optimize Energy Efficiency Without Compromising Comfort
Cheaper sensors, efficient data acquisition and processing systems, advancement in data-driven algorithms, and widespread adaptation of cloud computing have significantly enhanced how we optimize energy systems. These advancements are particularly impactful in building automation, as buildings consume 40% of total energy use in the United States. For mission-critical buildings, such as hospitals or data centers, maintaining strict constraints is essential. To meet these requirements, operators rely on building automation systems — complex networks of sensors, heating and cooling systems, air handler units, and centralized controllers.
Minimizing energy consumption in a large, dynamic system with hundreds of interconnected rooms is a highly complex challenge. This complexity stems from the need to accurately model time-varying system dynamics and dependencies across control variables — tasks that advanced ML algorithms exceed at. Indeed, in such systems, learning, control and optimization are inherently interconnected. The key to efficient operation lies in having a unified platform that seamlessly integrates these capabilities, enabling easy deployment, monitoring, and configuration.
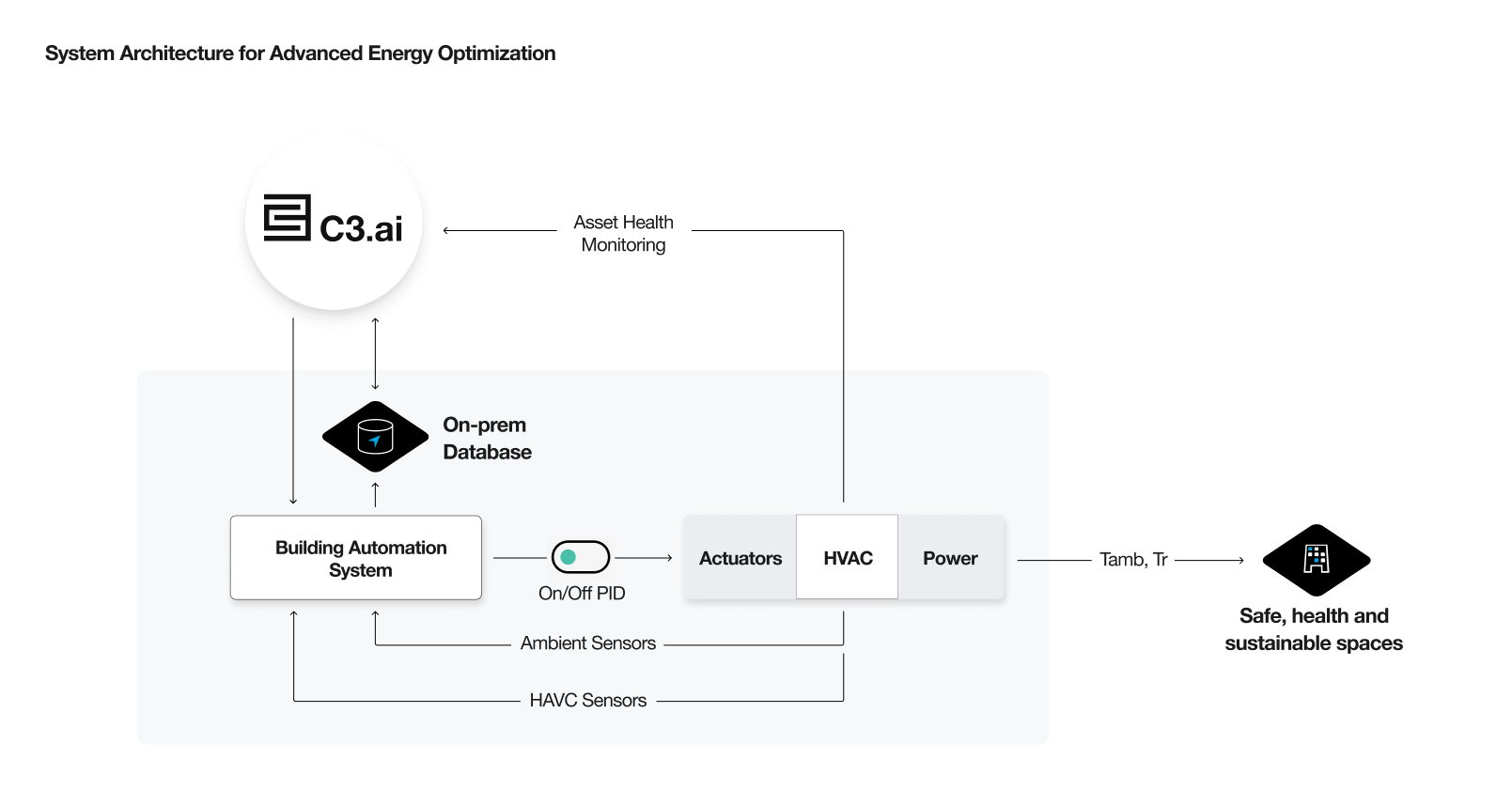
Figure 1: C3 AI’s system architecture optimizes HVAC energy use, reduces costs, and improves efficiency while maintaining indoor climate conditions.
Using the capabilities of the C3 AI Platform, we have developed and deployed a solution in under four months for a mission-critical facility in North America. This solution incorporated multiple ML models to predict physical variables such as humidity and temperature, alongside a mathematical optimization framework to determine optimal operating conditions and minimize total energy consumption. The solution is fully and easily integrated to the customer’s existing infrastructure, thanks to the model-driven architecture of the platform, which, consequently, accelerated the C3 AI Data Science team’s model development timeline. The overall solution architecture is illustrated in Figure 1.
Predict and Control Energy Use with AI
For end users wanting to optimize their systems, understanding the impact of their actions and the evolution of system states is invaluable. This is the core of the solution developed by the C3 AI Data Science team. By leveraging the model deployment and optimization framework of C3 AI Platform, the team captured the dynamic relationships between sensor measurements, control variables, setpoints, and the total energy consumption, enabling global minimization of energy use. A simplified mathematical framework is shown in Figure 2 where the goal is to minimize the total energy consumption across M rooms over a horizon of H while satisfying the user constraints.
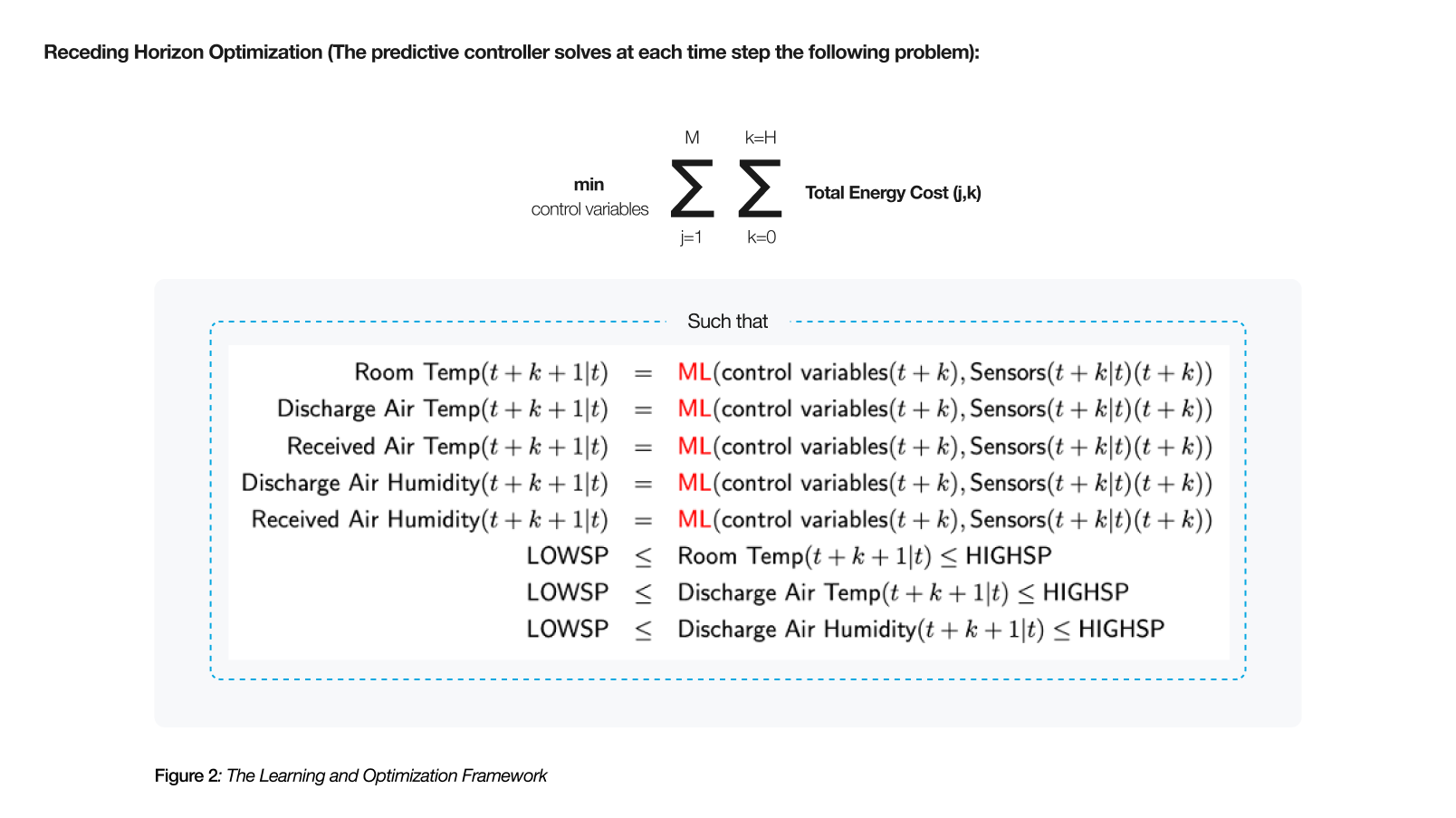
Figure 2: Machine learning-based framework for HVAC system optimization.
With accurate models predicting room temperature and humidity based on control actions, the solution generated optimal recommendations for set points to minimize total energy costs. These recommendations could be tailored to user-defined configurations, such as prioritizing reductions in electricity costs over natural gas costs.
This approach demonstrated significant real-world effectiveness, achieving over a 10% reduction in total energy costs by balancing natural gas and electricity costs.
This was accomplished by reducing natural gas consumption in steam boilers, increasing electricity consumption of the heat recovery chiller—which recovers heat after cooling the air—and slightly increasing natural gas consumption in hot water boilers. The results of this optimization are illustrated in Figures 3 and 4.
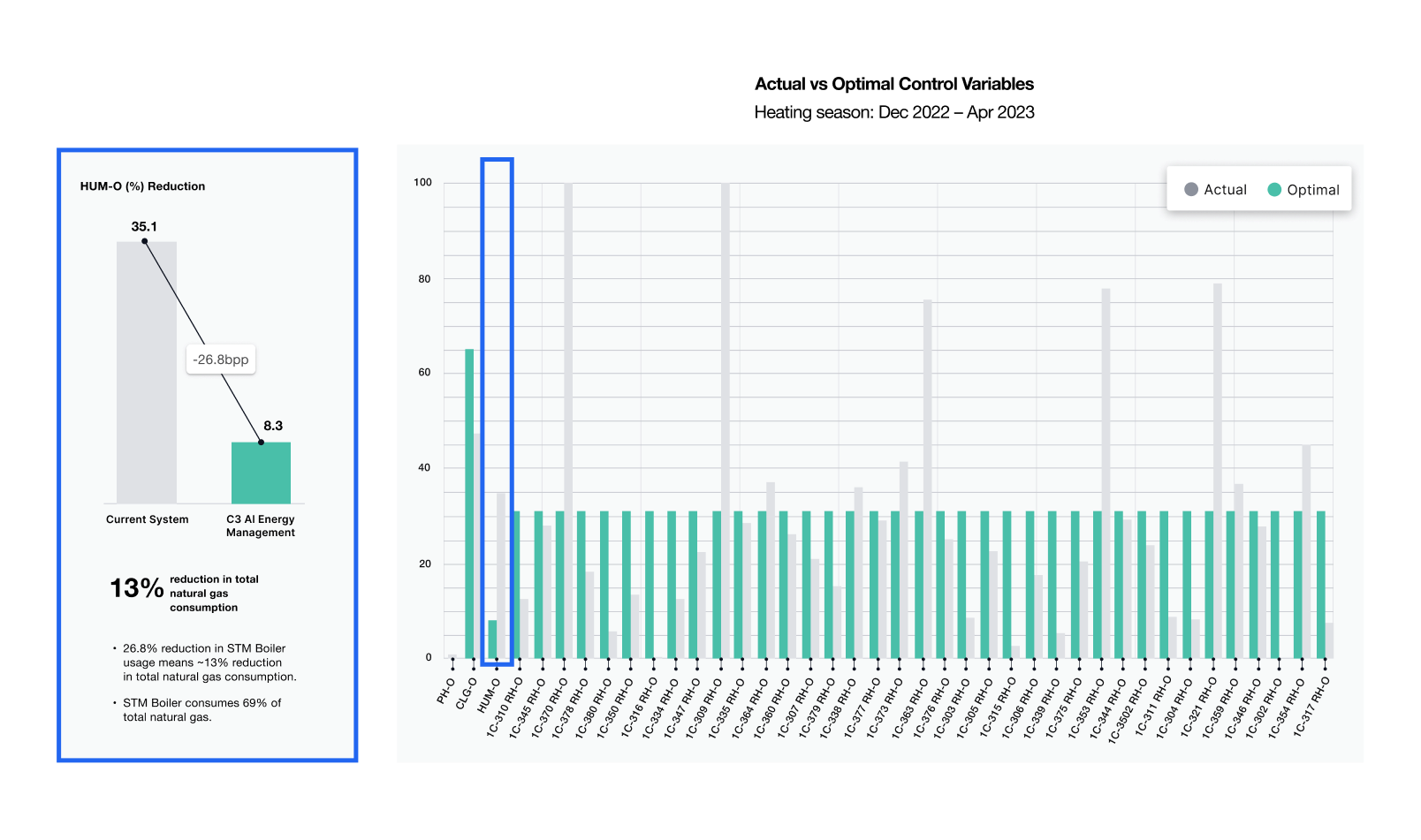
Figure 3: Optimizing steam boiler operations through the humidification valve (HUM-O) enables a projected 13% reduction in natural gas consumption and cost savings.
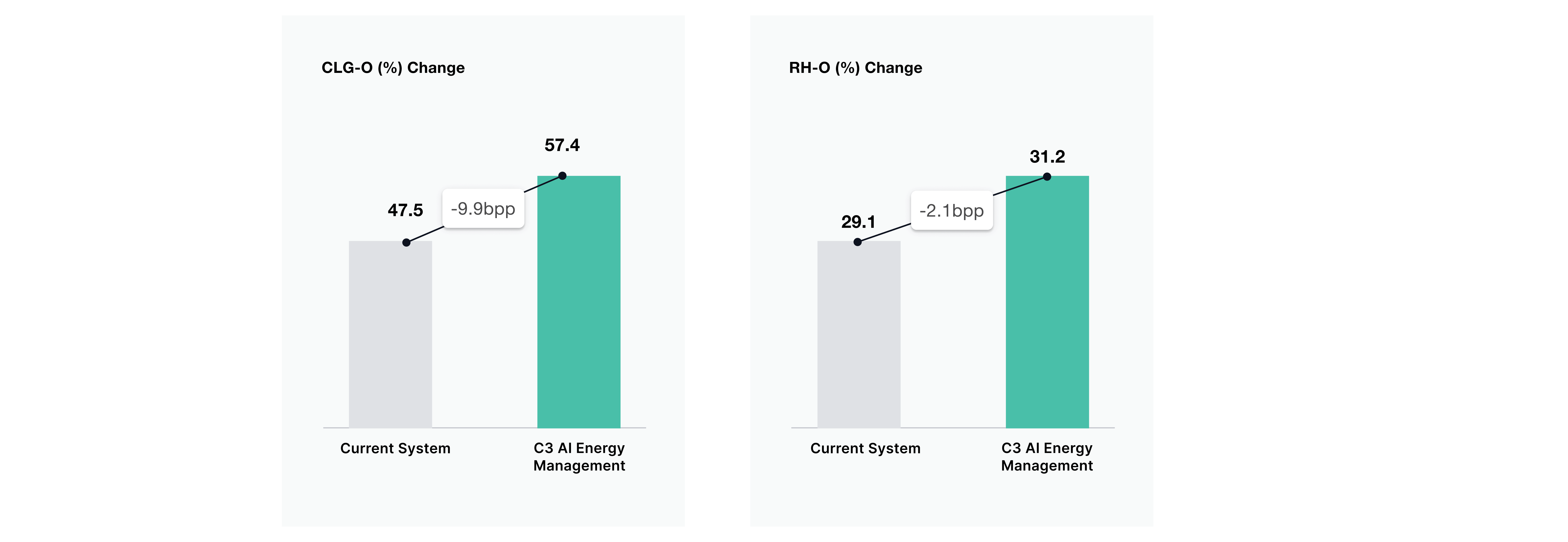
Figure 4: Increased heat recovery chiller and hot water boiler usage (via the cooling valve (CLG-O) and the reheating valve (RH-O) respectively) offsets reduced steam boiler consumption, balancing energy efficiency and environmental comfort.
Scaling Energy Optimization Across a Fleet of Buildings
HVAC and related system providers often manage thousands of buildings. Scaling an energy optimization solution from a single building to thousands requires a streamlined approach to deployment, monitoring, and maintenance. The challenges are significant, including accessing accurate, up-to-date data from diverse and asynchronous sources, developing high-quality ML models for many system components, ensuring near-real-time accuracy, and generating optimal operating conditions that balance data, learned models, and user requirements.
The C3 AI Platform simplifies this complex process, making it systematically manageable. The platform includes prebuilt, extendable data models that are easily configurable for new complex use cases, providing unified access to all relevant data through a simple SDK.
A critical step in scaling data-driven models is creating and selecting the input features that the models are trained on. The C3 AI Feature Store on the C3 AI Platform addresses this by enabling users to create, monitor, store, reuse, and share ML features across assets and buildings. With over 200 pre-built features, the Feature Store accelerates experimentation and application development while ensuring model reusability and scalability. Teams can define and store features along with metadata, then share those features through a central registry for broader use.
The C3 AI–hosted Jupyter Hub, fully integrated with the C3 AI Platform, further enhances scalability by providing seamless access data and simplifying ML model experimentation. Data scientists and subject matter experts can efficiently iterate and refine solutions using multiple data sources in a standardized environment.
Finally, the C3 AI Model Deployment Framework enables scalable deployment monitoring. Leveraging the platform’s elastic, multi-node architecture, thousands of models can be trained, processed, and tuned simultaneously using asynchronous compute jobs. The framework allows users to easily configure data training logic, ensuring flexibility across systems. Take air handler units for example: for this system, training data can be shared across many units in a building or kept specific to individual ones.
A Unified AI Platform for Smarter Energy Management
Access to data from thousands of internal and external sensors unlocks opportunities far beyond optimizing operating conditions. By improving the efficiency and overall health of building assets, energy consumption can be further reduced while extending system longevity.
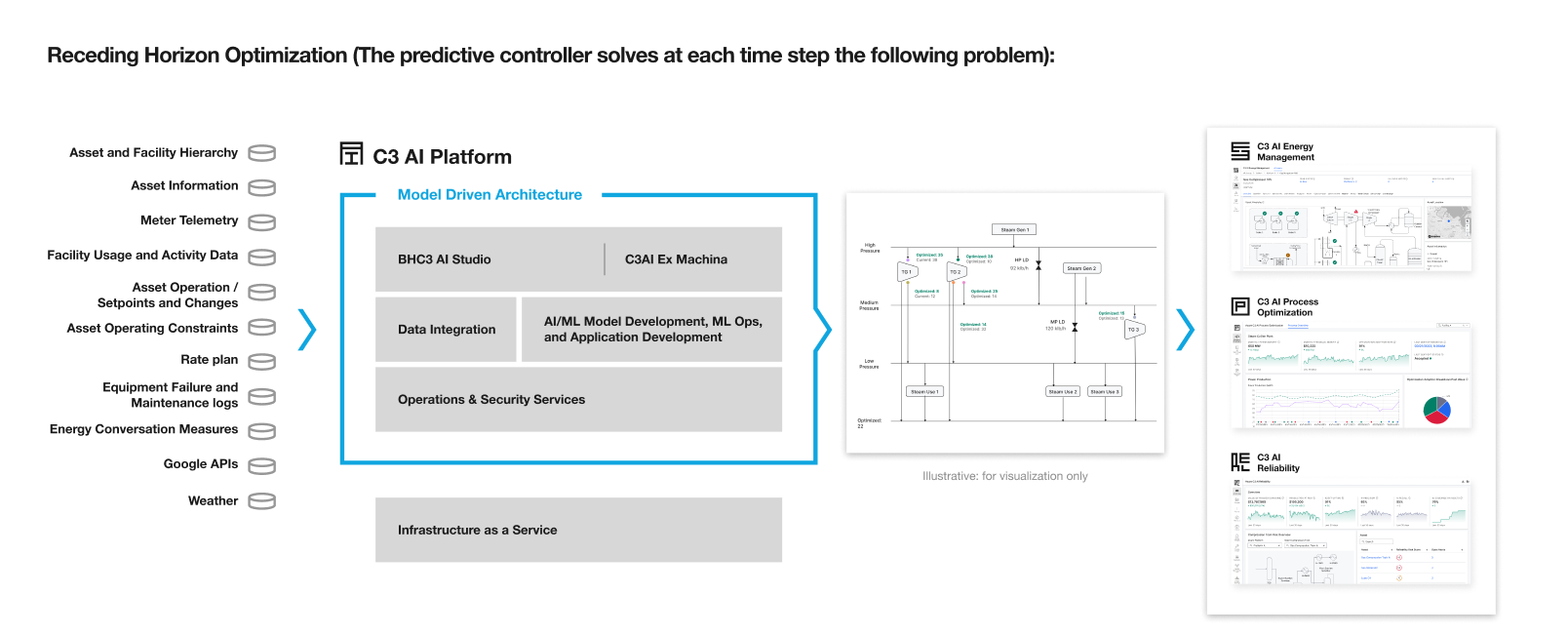
Figure 5: The intelligent energy management system unifies AI-driven optimization to cut costs, improve reliability, and extend asset life.
Using the unified data model capabilities of the C3 AI Platform, data scientists developed additional ML models to predict inefficiencies in assets like chillers. These models enable proactive maintenance, allowing users to address issues before they escalate and run the systems more efficiently for longer periods. The team successfully demonstrated the ability to predict and prevent catastrophic failures weeks before occurrence. Figure 6 illustrates this capability, showing the C3 AI Reliability risk model’s output providing accurate early warnings of potential risks.
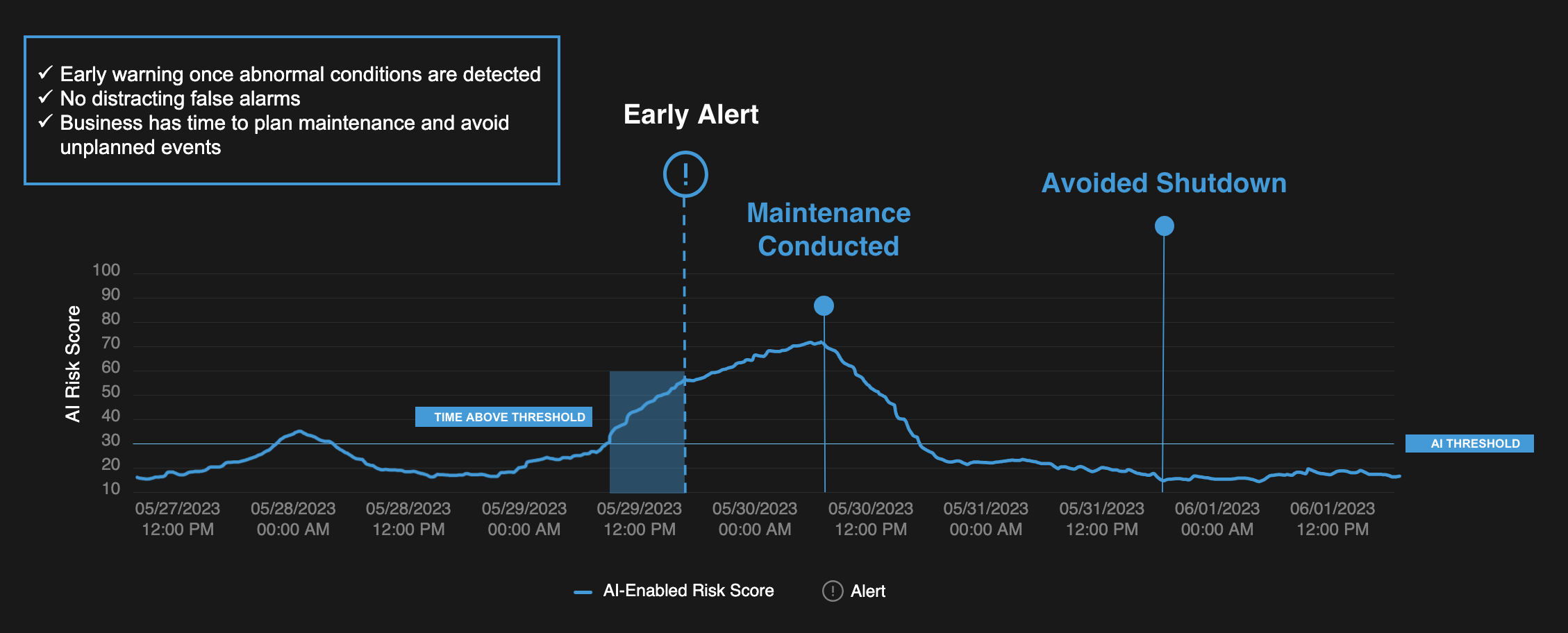
Figure 6: AI-based monitoring predicts potential failures early, using real-time data to reduce downtime and prevent costly disruptions.
This unified approach to energy management not only reduces costs but also ensures more efficient, resilient operations at scale.
Ready to transform your energy strategy? Discover how the C3 AI Platform can help your organization achieve unparalleled energy efficiency and operational resilience.
About the Authors
Nevroz Sen is a Data Science Manager at C3 AI. He holds a Ph.D. degree in Applied Mathematics from Queen’s University, Canada. Previously, he held post-doc positions at Harvard University and McGill University and worked as a principal engineer in the areas of machine learning and robotics. His research interests include machine learning, information theory, stochastic control, mean field games and nonlinear filtering.
Mehdi Maasoumy is a Vice President of Data Science at C3 AI where he leads AI teams that develop machine learning, deep learning, and optimization algorithms to solve business problems including stochastic optimization of supply chains, reliability, sustainability, and energy management solutions across industries. Mehdi holds a PhD from the University of California at Berkeley in Optimization and Machine Learning. He has authored more than 50 peer-reviewed papers and books in machine learning and optimal control and is the recipient of three best paper awards from ACM and IEEE.
Naoufal Layad is a Senior Data Scientist at C3 AI, leading the development of the Energy Management application. He holds an M.S. in Computational and Mathematical Engineering from Stanford University and dual engineering degrees from Paris-Saclay University (CentraleSupélec, France). Previously, he conducted research at SLAC National Accelerator Laboratory, where he developed AI models for high-energy physics applications. His work centers on advancing AI-driven energy optimization and sustainability solutions.