Law enforcement agencies in the U.S. are grappling with rising crime rates, including a 12% spike in violent crime in the last decade. Although many factors contribute to this rise, a few stand out: the COVID-19 pandemic, economic insecurity, and a lack of trust between police and the communities they serve. In general, government agencies are slow to adopt new technologies that can improve public safety for a variety of reasons including growing community concerns over privacy violations and lack of oversight.
Law enforcement agencies need transparent, auditable, and ethical systems to effectively fight crime while ensuring trust of the communities they serve in securing public safety. Now, many agencies are looking towards better integrating technology into their decision-making processes, applying analytics to large amounts of data, and optimizing processes to improve efficiency and public trust. By combining data fusion and artificial intelligence (AI) to enhance investigations, agencies can foster safer communities and ultimately attain a higher level of public trust.
Analysts and investigators often work with outdated, manual processes that are prone to error and time consuming. Law enforcement analysts and investigators often need to work with disparate sources of information and these practices can result in investigators missing critical facts or making factually incorrect assessments, which jeopardizes the public’s trust. When a typical workflow involves manually consolidating data from internal and external sources including county systems, dispatch systems, license plate readers, and incarceration data, long investigative timelines and bottlenecks are normal.
Increasing Collaboration and Communication with Data Fusion
Many criminals have histories across counties and states, but most law enforcement agencies can only access their own records. One solution to this problem is to unify and standardize data across multiple agencies, giving law enforcement easy access to a suspect’s complete criminal history. In fact, in response to 9/11, the U.S. government started this data sharing process and began setting up data fusion centers to increase collaboration, communication, and information sharing across agencies. These state-owned centralization bodies fuse intelligence from across different agencies that include state, local, tribal, federal, and private sector partners.
Today, many of these same contributing agencies are also working to establish in-house data fusion capabilities. To achieve that, not only does each agency need internal and external investigative sources fused, but also data from neighboring jurisdictions.
Traditional methods of data sharing are antiquated and time consuming — but using AI can accelerate data cleaning and consolidation at scale. The same auto-correct technology that most people use daily while typing or texting can be leveraged to increase the accuracy of suspect input and report writing — a typo may be the difference between finding a lead from years prior and letting a case go unsolved. Additionally, as gaps in the data exist between systems, the central layer between them must automatically reconcile these gaps rather than waiting for a person to manually merge the data.
For example, if one agency collects data on a suspect’s whereabouts and another agency has information on the suspect’s associates, combining these two data sets can help investigators build a more complete profile of the suspect’s history and patterns.
Data fusion also unlocks more powerful AI-driven insights. The more data machine learning models have access to, the better the predictions. Having more data to learn from can help the system identify more complex patterns and relationships, which feeds into more informed policing.
For instance, if two neighboring counties are experiencing an increase in thefts from vehicles, their shared data platform could use machine learning algorithms to identify patterns in the data, such as the time of day or location of the thefts, the type of vehicle targeted, or any other common factors. This intelligence could then be used to develop targeted enforcement strategies or public education campaigns aimed at reducing these types of crimes.
The same principle applies to suspect behavior. Agencies with advanced data fusion capabilities can develop a more comprehensive understanding of a criminal’s behavior and movements, increasing the likelihood of apprehension. This approach can also be more efficient and cost effective than each agency working independently, as it minimizes duplicative efforts and allows agencies to leverage the expertise and resources of their counterparts in other counties.
A Pre-Built AI Solution for Law Enforcement
To enhance public safety and in support of law enforcement agencies and the challenges they face, C3 AI developed an integrated, AI-powered intelligence analysis platform. The C3 AI Law Enforcement application enables analysts, investigators, and uniformed officers to efficiently aggregate, access, explore, and manage otherwise disparate structured and unstructured datasets to derive investigative insights in near real time.
In addition to unifying the data, C3 AI Law Enforcement provides a dashboard with jurisdiction-wide crime trends and geospatial views, a search capability that checks all ingested systems simultaneously to display rapid results, a blackboard that explores relationships between identities based on a variety of relationship types, identity pages that pull from every data source to create a singular summary of suspect information, and a case management functionality that enabled interacting with criminal offenses and finding related identities.
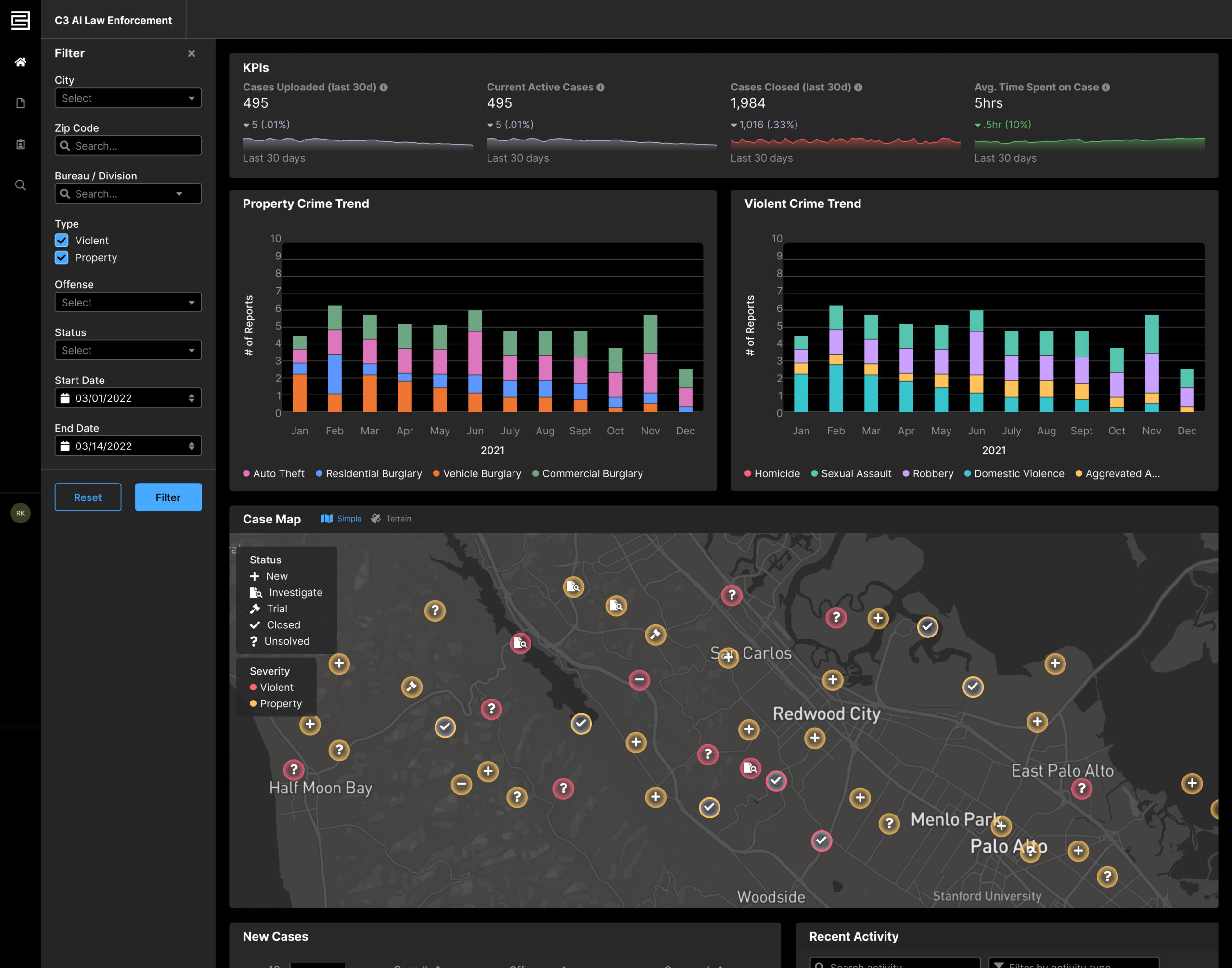
C3 AI Law Enforcement Application Dashboard
C3 AI Law Enforcement is currently being used by law enforcement agencies in the US. These agencies face many of the same common challenges — multiple disparate data sources, diverging data, and a siloed IT solution stack.
With C3 AI Law Enforcement, agencies have seen significant benefits including:
- A centralized database: Disparate investigative data sources ingested and contextualized into a unified data image
- Organized, de-duplicated records: Hundreds of thousands of records linked across disparate data sources
- Faster, easier access to statistics and crime trends: Turnaround times decrease from an average of four days to instant availability
- Faster, easier access to suspect history: Information about suspects that traditionally takes hours to compile becomes readily available
Following the initial deployment of the C3 AI Law Enforcement application within US law enforcement agencies, project teams are incorporating data collaboration across jurisdictions to bring in the benefits of data fusions between agencies, among many other features and data enhancements. As functionality is developed and neighboring agencies participate, the benefits will continue to accumulate.
Grant Guttschow is a Senior Product Manager at C3 AI focused on Defense & Intelligence applications, including C3 AI Law Enforcement. Grant holds academic degrees from the United States Naval Academy and the University of Illinois Urbana-Champaign. Previously, Grant served as an active-duty Marine Corps officer, specializing in cyberspace and information operations.