A guide for supply chain leaders investing in AI solutions
By Anna Ershova, Director of Products, C3 AI and Vivek Bhushan, AI Solution Director, C3 AI
Artificial intelligence (AI) is revolutionizing the supply chain industry with transformative solutions leading to more accurate demand forecasting, inventory optimization, and improved customer order fulfillment and service. The AI-powered applications available today — such as those in the C3 AI Supply Chain Suite — are far different from the solutions from the past.
Although the emergence of generative AI in the mainstream is a cause for great excitement for supply chain leaders, many are unsure about exactly what generative AI can (and cannot) do to help build more resilient supply chains.
The common generative AI narrative we often hear is that it can solve all forecasting, optimization, and analytical challenges in the supply chain space: ChatGPT will tell users that generative AI can add the most value in demand forecasting, inventory optimization, and supply chain simulation. However, traditional AI — not generative AI — has already addressed these challenges well.
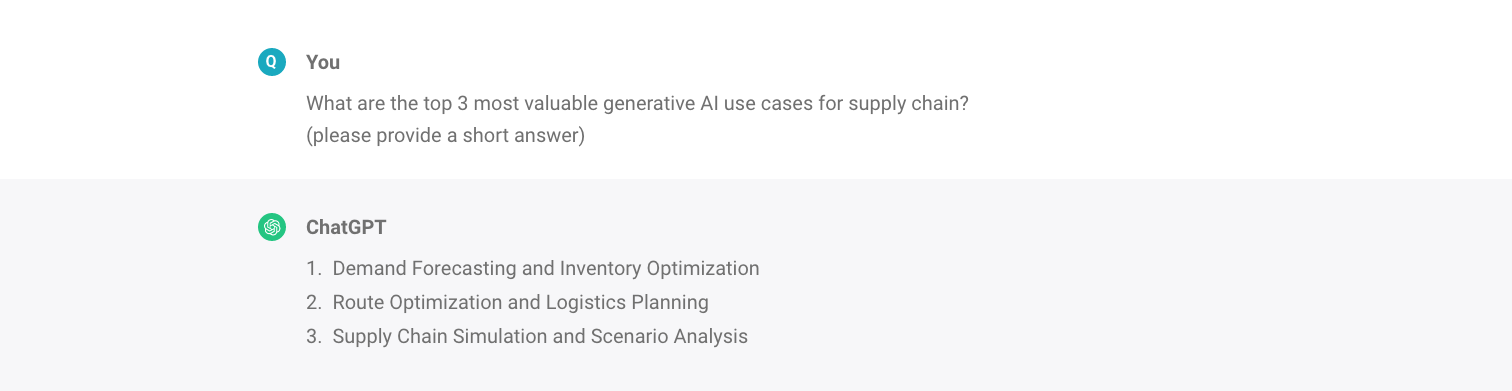
ChatGPT answers a question about how generative AI can add value to supply chain management.
As technology advances, consumers must be able to distinguish between the two primary AI paradigms shaping the future of supply chains: traditional AI and generative AI. Each offers unique benefits and caters to different aspects of supply chain management, so the question remains: what are the differences and where should they be applied for the best return on investment?
Traditional AI for Supply Chain: The Predictive Powerhouse
Traditional AI is the subset of AI that utilizes machine learning (ML) algorithms to enable systems to learn from data and make predictions or decisions without being explicitly programmed for every task.
Traditional AI has become a cornerstone for the supply chain, mainly due to its predictive analytics and optimization capabilities. Its algorithms are highly effective at forecasting demand, right-sizing inventory, optimizing production planning and schedules, and predicting transportation delays. By utilizing historical and real-time data from both internal and external sources to train ML models, traditional AI provides businesses with actionable insights to make informed decisions ahead of time.
For example, in demand forecasting, complex ML algorithms can be leveraged to analyze internal and external data to offer more accurate demand predictions than traditional statistical methods. Traditional AI also helps businesses predict supply and demand uncertainties in inventory optimization. This results in minimized holding costs, fewer stock-outs, and better inventory turnover while maintaining the promised service levels.
Generative AI for Supply Chain: The AI Democratization Catalyst
Generative AI represents a more advanced stage of AI development, creating systems capable of autonomously generating new content, ideas, or solutions.
Generative AI is transforming how we interact with the data within the supply chain. Large language models (LLMs) are built to recognize, summarize, translate, and generate content. Generative AI democratizes and expedites access to data within an organization.
A knowledge assistant is a typical generative AI supply chain use case. Through a natural language interface, users can ask complex questions about their data that would otherwise take a long time and many hours to answer because of the lengthy data synthesis required.
Another significant use case is interpreting ML model results. Many supply chain teams struggle to trust black-box ML models that provide no explanation of their outputs. In contrast, C3 AI applications supplement each ML model with an evidence package. Generative AI enables even non-technical users to interact with the model. For example, demand planners can use it to quickly uncover what drove a change in the AI demand forecast, how drivers affecting a forecast are different from one another, or discover hidden demand trend changes. This opens opportunities for broader workforce engagement in data-driven decision making.
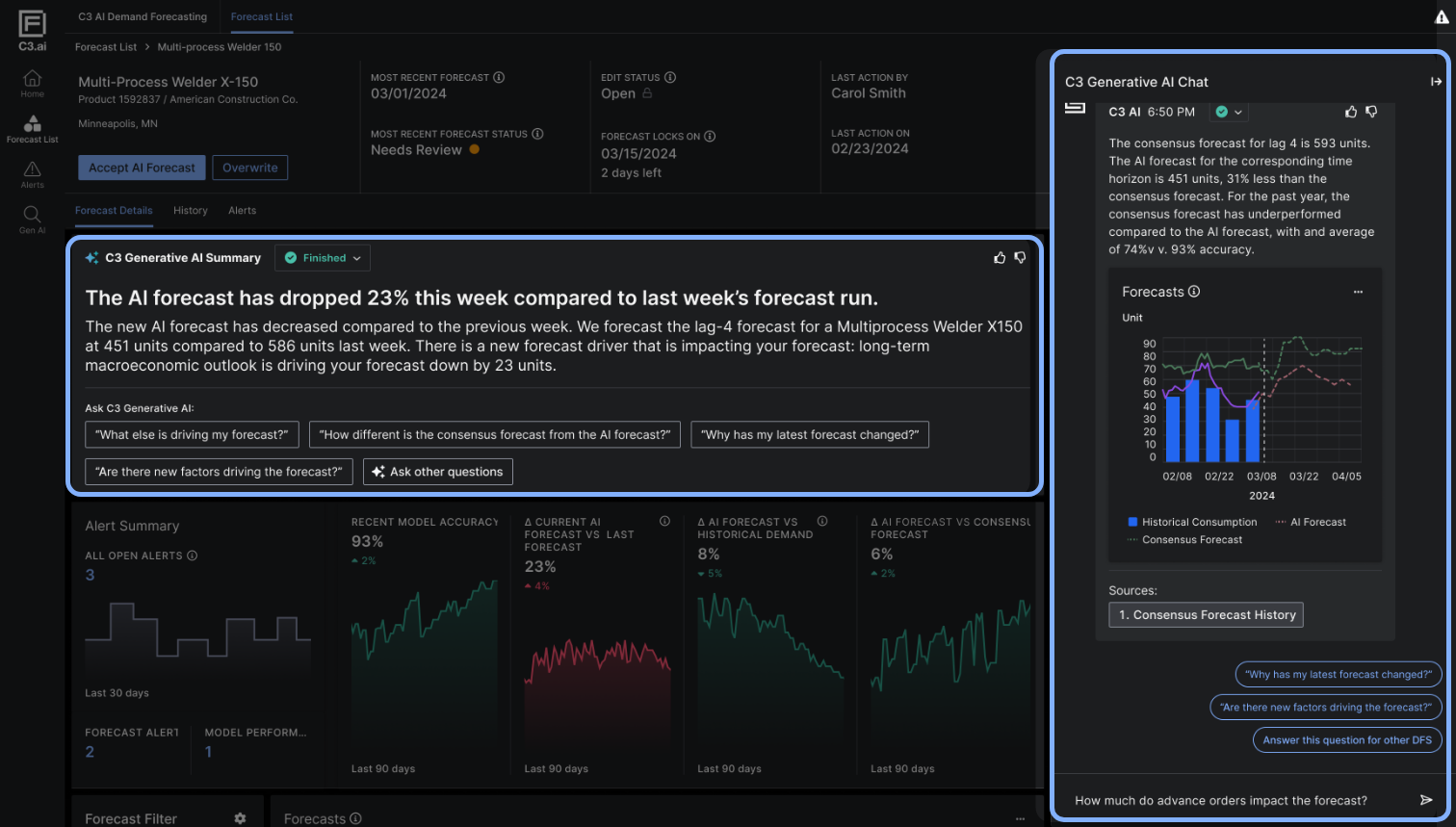
C3 Generative AI explains why the AI demand forecast dropped significantly to supply chain teams through interactive chat.
However, generative AI is not optimally designed to perform predictions, optimization, or what-if simulation, all of which require analyzing large amounts of structured data (e.g., purchase orders, pricing, sales history). While training LLMs to perform those use cases is technically feasible, it requires significant investment in fine-tuning to achieve the effectiveness level that can already be obtained with traditional AI at lower cost and effort levels.
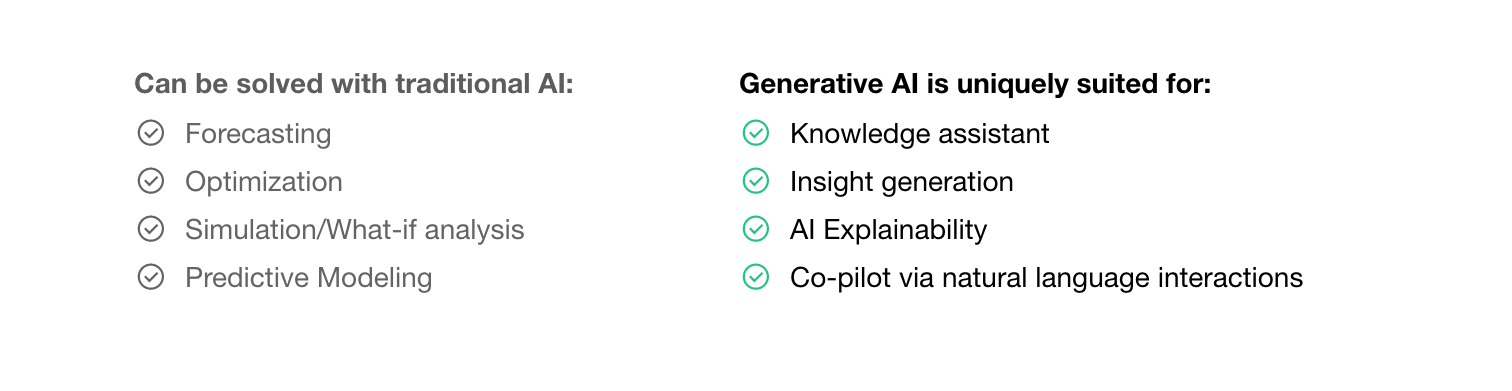
Traditional AI and generative AI provide different solutions within the supply chain sector.
Secure a Competitive Edge with the Best of Both Traditional AI and Generative AI
Many supply chain leaders are wrestling with how to be at the forefront of the generative AI revolution. Neither generative AI nor traditional AI is the silver-bullet answer. Combining advantages of both will maximize AI’s potential, improve adoption, and build competitive advantages for supply chains.
Supply chain organizations should start with building a unified system of enterprise data and external data sources to provide end-to-end visibility across the supply chain and create a foundation for developing AI models. Then, traditional AI models can be built to give predictions and provide optimization and automation to help the supply chain team make more accurate and proactive decisions. Finally, generative AI provides rapid and intuitive access to critical, relevant insights and predictions for everyone in the supply chain team, enabling faster decisions at all levels.

Generative AI combined with traditional AI delivers the most value to supply chain organizations.
For example, many supply chain leaders want to proactively manage supply network risks and build resiliency plans for potential disruption. To do that, the first step is to unify all the data across the supply chain. Then, lead time prediction models and scenario modeling capabilities using traditional AI are built to help supply chain leaders know which orders are at risk and how to react. Finally, generative AI enables supply chain teams to simply ask: “What orders are at risk and why?” instead of performing multiple, manual analyses.
How C3 AI Can Deliver Value for Supply Chain Leaders
While traditional AI continues to serve as the predictive and optimization engine of supply chains, generative AI is reshaping how we interact with and analyze data. Both are invaluable to the supply chain sector.
We recommend that supply chain entities use both technologies to create a robust, responsive, and intelligent supply chain ecosystem capable of withstanding the uncertainties of the modern business world.
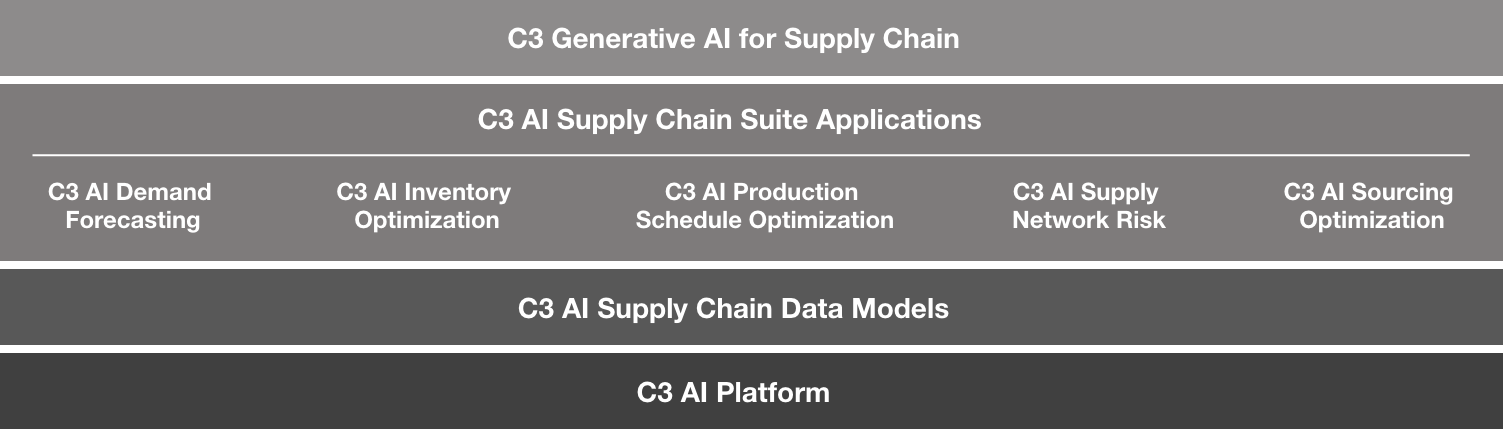
C3 AI offers a complete suite of products for supply chain leaders to build successful enterprise AI programs.
The C3 AI Supply Chain Suite is the best partner for supply chain organizations to build competitive edges by leveraging traditional AI and generative AI. Our solutions include unified supply chain data models, supply chain applications — C3 AI Demand Forecasting, C3 AI Inventory Optimization, C3 AI Production Schedule Optimization, C3 AI Supply Network Risk, and C3 AI Sourcing Optimization — and C3 Generative AI for Supply Chain. We bring the best of both worlds with best-in-class traditional AI models and high-value generative AI solutions built specifically for the supply chain.
About the Authors
Anna Ershova is a Director of Supply Chain Products at C3 AI where she leads the development of C3 AI Supply Chain Suite of applications. She has 10+ years of experience building, deploying, and scaling leading AI software for large enterprises.
Vivek Bhushan is an AI Solution Director at C3 AI with over a decade of experience at the intersection of supply chain strategy, AI, and technology across various industries.